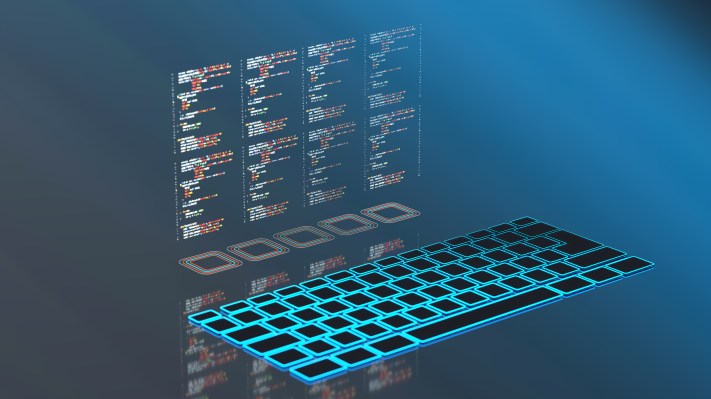
Of all enterprise departments, product and engineering spend by far the most on AI technology. Doing so effectively stands to generate huge value — developers can complete certain tasks up to 50% faster with generative AI, according to McKinsey.
But that’s not as easy as just throwing money at AI and hoping for the best. Enterprises need to understand how much to budget into AI tools, how to weigh the benefits of AI versus new recruits, and how to ensure their training is on point. A recent study also found that who is using AI tools is a critical business decision, as less experienced developers get far more benefits out of AI than experienced ones.
Not making these calculations could lead to lackluster initiatives, a wasted budget and even a loss of staff.
At Waydev, we’ve spent the past year experimenting on the best way to use generative AI in our own software development processes, developing AI products, and measuring the success of AI tools in software teams. This is what we’ve learned on how enterprises need to prepare for a serious AI investment in software development.
Carry out a proof of concept
Many AI tools emerging today for engineering teams are based on completely new technology, so you will need to do much of the integration, onboarding and training work in-house.
When your CIO is deciding whether to spend your budget on more hires or on AI development tools, you first need to carry out a proof of concept. Our enterprise customers who are adding AI tools to their engineering teams are doing a proof of concept to establish whether the AI is generating tangible value — and how much. This step is important not only in justifying budget allocation but also in promoting acceptance across the team.
The first step is to specify what you’re looking to improve within the engineering team. Is it code security, velocity, or developer well-being? Then use an engineering management platform (EMP) or software engineering intelligence platform (SEIP) to track whether your adoption of AI is moving the needle on those variables. The metrics can vary: You may be tracking speed using cycle time, sprint time or the planned-to-done ratio. Did the number of failures or incidents decrease? Has developer experience been improving? Always include value tracking metrics to ensure that standards aren’t dropping.
Make sure you’re assessing outcomes across a variety of tasks. Don’t restrict the proof of concept to a specific coding stage or project; use it across diverse functions to see the AI tools perform better under different scenarios and with coders of different skills and job roles.